Quality Engineering, Digital Employees and Job Security
Is Generative AI going to take our jobs?
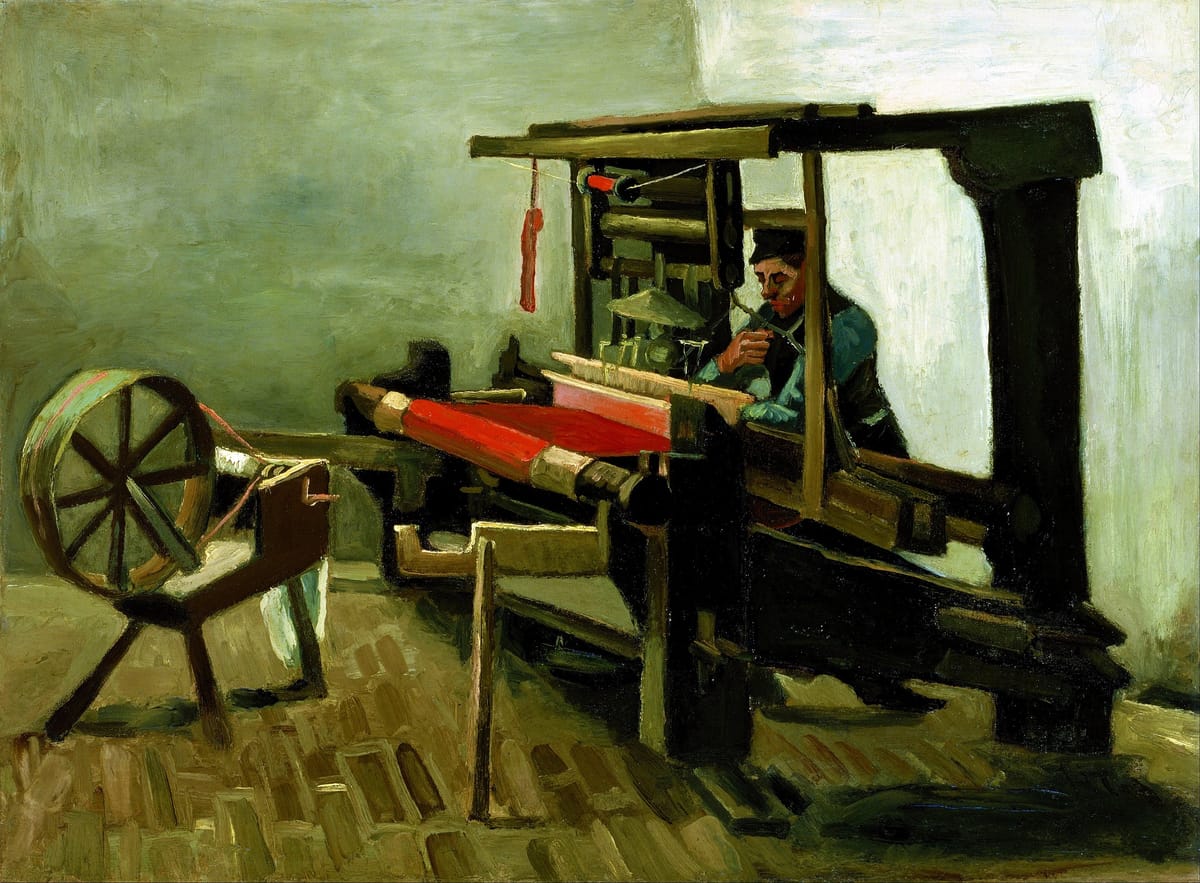
Whilst a lot of focus has been on the drama of Crowdstrike in the past week, my attention has been drawn towards the conversations around Digital Employees, I.E: AI-based Agents. The debate started when the CEO of Lattice shared a blog and social media posts about being one of the first companies to give Digital Employees official company records. The reaction was mixed at best. However, it got me thinking more about the impact of AI on quality engineering roles in the future. What’s in store for us?
I can always sense a low level of anxiety when I am asked about the future of testing in a world where we have generative AI. The common response is “It won’t take our jobs, but it will change them”. But what does that mean exactly? And are we entirely convinced by that claim? Are we at risk? How much change is in the air? Should we be worried? I’ve been watching webinars, experimenting with features and taking part in a fair few trials with generative AI and these are a few thoughts on what might be in store for us.
Gen AI is a piece of the continuous testing puzzle
Recently I attended Applitools’ webinar on the release of their Autonomous 2.0 service, their latest offering in Generative AI-backed testing. I must admit I was a little underwhelmed. It’s not to say that what Applitools is offering isn’t technically impressive. It’s by far the most advanced codeless automation tool I’ve seen so far. But, what leaves me cold is the scope of a tool that calls itself autonomous. When I think of autonomous testing I, and most likely others, are imagining AI Agents that are capable of providing value across a wide spectrum of activities in which a quality engineer is involved.
So far testing tools backed by Generative AI that have been released have been mostly focused on generating test cases. This is likely unsurprising for some as the majority of testing tools are rooted in the test case space. But if we consider where Gen AI is being targeted in the wider scope of continuous testing we begin to appreciate that there is a lot left untouched that we are responsible for. To put it another way, consider the continuous testing model Dan Ashby in his blog Continuous Testing in DevOps…
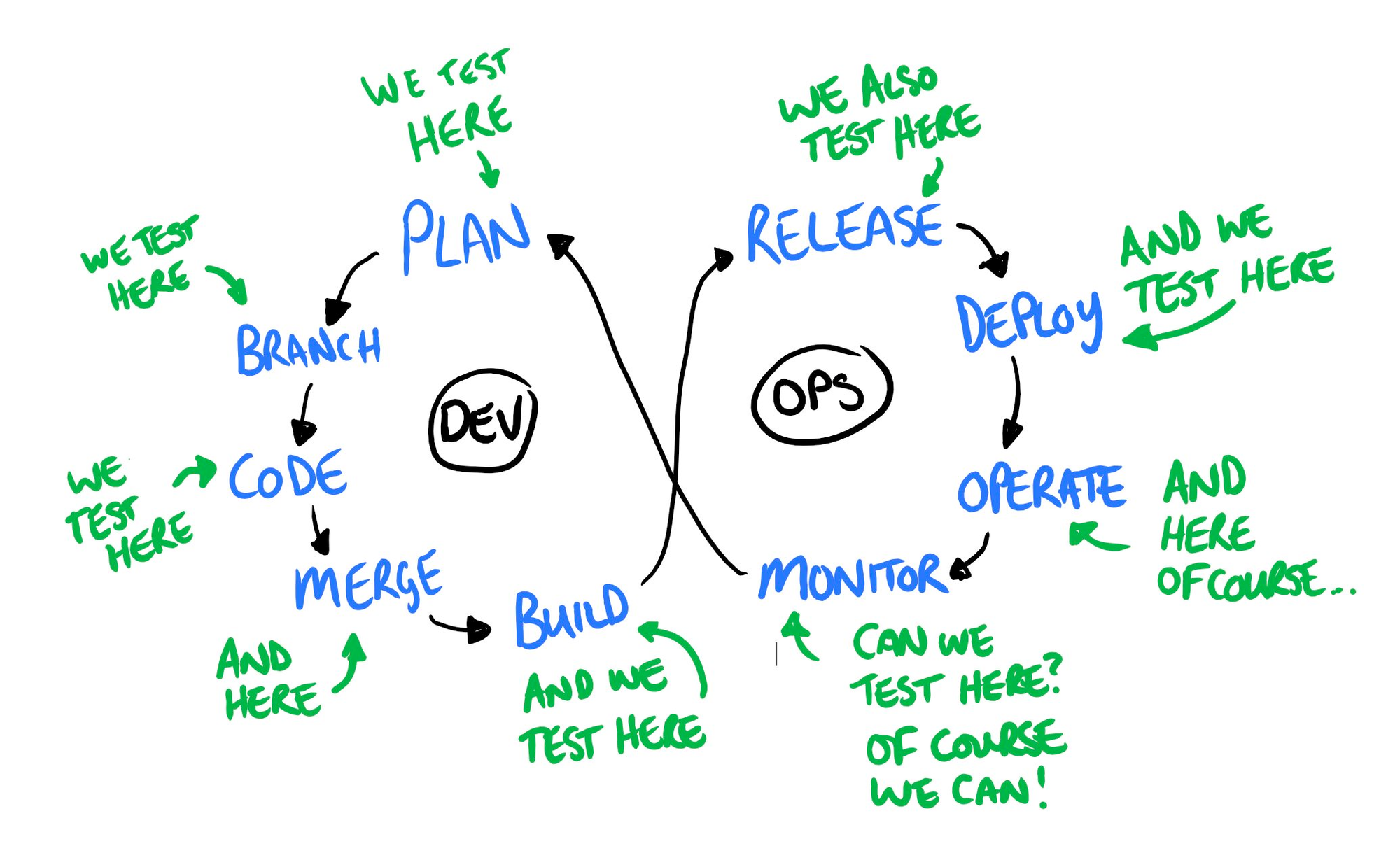
Dan’s model expertly communicates that we are involved in many facets of the development process. Now let’s add the focus of current, popular, Gen AI tools into the model to see what they are focused on:
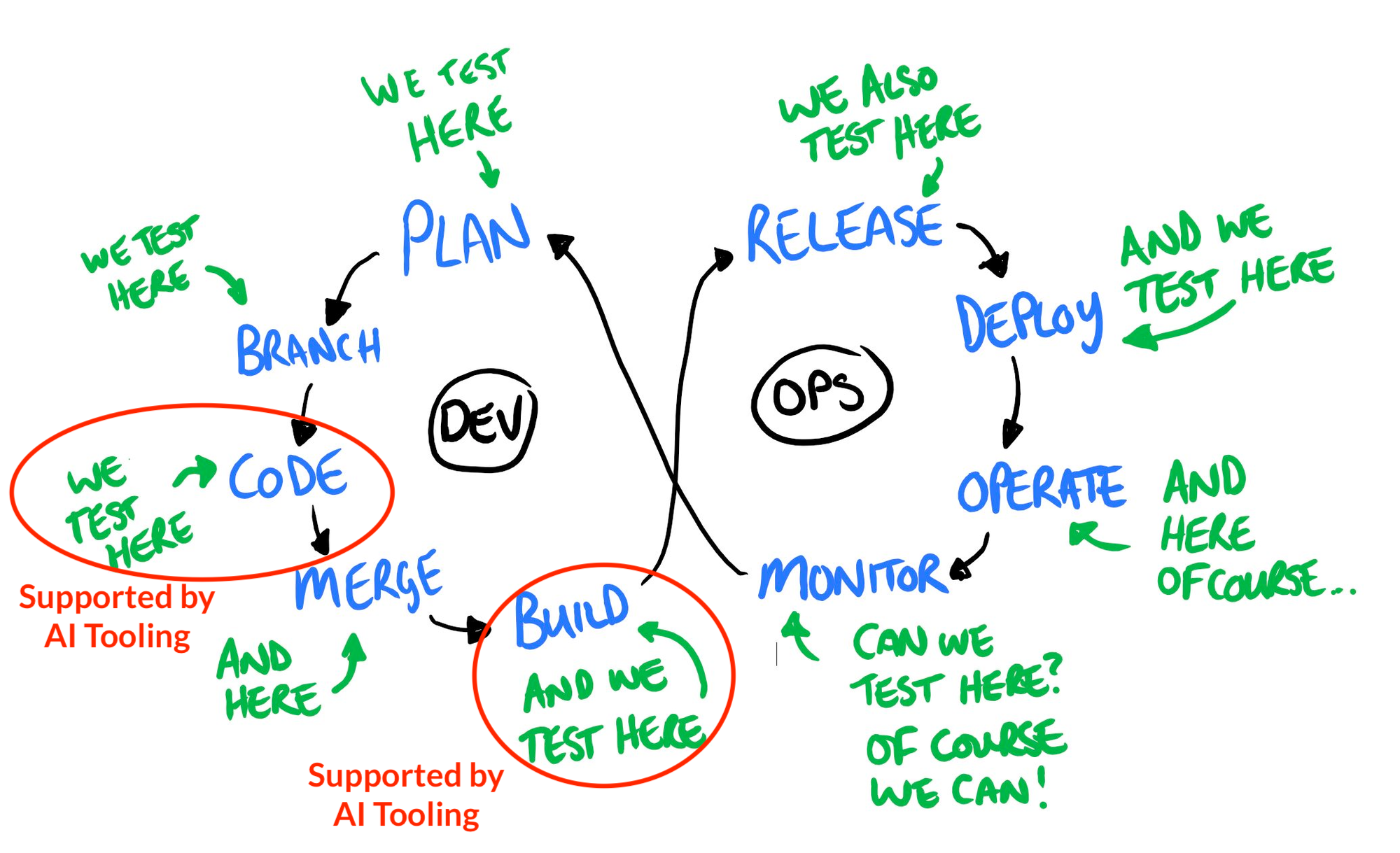
As we can see, they are very much rooted in the code and build spaces. There is still so much across the lifecycle which a quality engineer can help with.
This argument, I believe, applies to test automation in general. Regardless of Gen AI technology, the prevailing perception of testing and quality is based too much on the build phase of a lifecycle (Hence why Dan wrote is original post). But if the majority of tooling and training is focused on that area, it squeezes out the other valuable activities. So when a new tool comes along claiming it’s automating testing / doing away with manual testing, some of us believe that to be the case because we’re not considering all the other ways in which we support teams.
That said, this is not to say that Gen AI cannot support us in those other areas of testing. It’s just that’s not where the money or interest lies. One of the reasons I wrote AI-Assisted Testing was to demonstrate how Gen AI can be used, with the right techniques, context and mindset, to support continuous testing activities. Perhaps we will see more tools appear in the future that help us with planning or monitoring. But for now, unless there are companies out there that are looking at the holistic whole of continuous testing, then it’s likely that Gen AI will only help us in patches.
Deterministic is AI-generated, Indeterministic is human-focused
Another interesting thought that has crossed my mind, as I reflect on these Gen AI-backed testing tools, is that if these companies are interested in incorporating Gen AI, then other non-testing tool companies must be as well. What I mean by this is just as Gen AI is making it easier to generate test cases to test deterministic products, the products of the future are moving towards using AI themselves making them more indeterministic. This means the paradigm of quality engineering has to change to accommodate indeterministic products. Which will likely squeeze out the need for test cases
I recently wrote about an AI Hackathon I participated in. Since then I’ve attended another and both involved teams sharing a diverse range of ways in which Gen AI might be used to help internal and end users alike. The use of AI is becoming more prevalent in software development, whether it’s Gen AI, Machine Learning or other areas of AI. This means not only quality engineers, but everyone in a team will need to learn new skills, techniques, models, etc. to build quality AI-driven products.
This makes me think that Generative AI may have more of a transformative role for us via the adoption of said technologies within our organisations. Just as these testing tools that claim to have automated the testing role appear, the game changes. This means our job security is rooted in our ability to evolve and learn new skills rather than defend against the rise of digital employees/
We still don’t know what Gen AI can do
Putting the Gartner Hype Cycle aside for one minute. We, as an industry, are still figuring out what Gen AI is useful for. We’ve seen the gold rush of companies wanting to sell AI as a service. Building a few prompts and sticking a UI on it, but that time seems to have passed (or at least we’re getting wise to it). If there’s one thing I’ve learnt working on delivering products is that the expectation of a product and the reality of it are two different things. The potential of Gen AI is large, but will the reality match? I saw this in the Hackathons we ran and the tools I’ve experimented with myself. Building a proof-of-concept application that is backed with Gen AI is one thing, but turning it into a mature, successful, product is another. It might seem weird for me to say this after writing a book on using Gen AI in testing, but AI-Assisted Testing is an exploration of the topic and its potential. Ultimately, it’s hard to make claims about its impact on our roles when we still don’t know what all of its benefits are, as well as its limitations.
We have bigger contributors to job losses
Finally, and perhaps most importantly, we humans are doing a pretty good job of creating job losses without the use of AI. What I. Currently, we’re facing a phase of stagnation within the technology sector and it’s easy to fall into the trap of assuming the automation of our roles is what is at the heart of it.
To get a sense of what is contributing to job losses I went through the list of redundancies listed in TechCrunch’s ‘Comprehensive list of 2024 tech layoffs’ as of January to July 2024. I read through the list and sought to capture the figures and reasons for why redundancies appeared. I grouped them into the following categories
- Administration - The company went bust / ran out of money.
- Cost Saving - The company needs to return to profit. AI could be utilised to replace a worker with a digital employee, but companies that are struggling financially aren’t necessarily in a position to take on new untested tooling when budgets are squeezed.
- Funding loss - Similar to cost saving, but the reason is a withdrawal of investment. Applies more to startups.
- Pivoting - A refocusing of services/products. Jobs are lost because they are no longer relevant to the new focus.
- Restructure - Reorganising to either free up cash flow for new growth areas or reduce bureaucracy within an organisation. Cash flow isn’t the issue. This is where we are most likely to see redundancies in light of AI tools. But also restructuring is used as PR to hide cost-saving activities.
This, of course, is anecdotal information, but I still feel there are trends worth discussing. This was what I discovered:
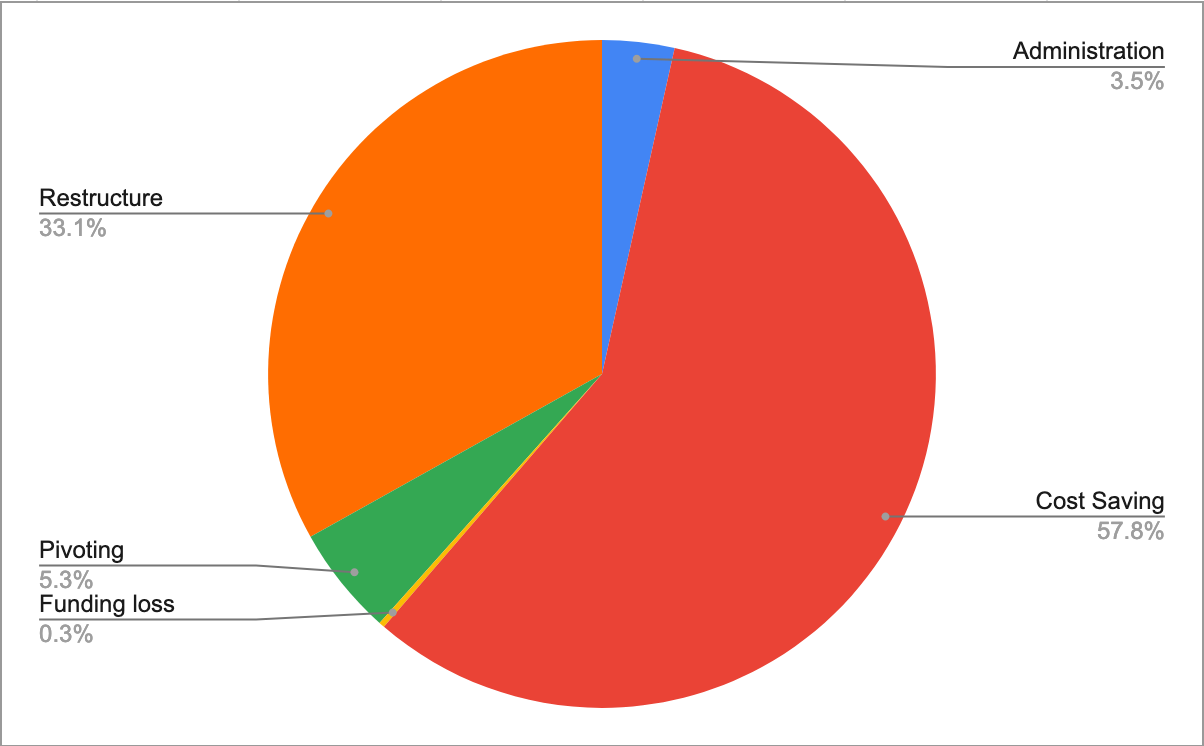
Over 50% of the reason for redundancies in companies was down to cash flow. Either they were not making enough money to begin with, or their operation costs swallowed their profit. A third of the companies wanted to restructure because they wanted to focus their attention on specific markets or they had simply hired too many people. These types of redundancies aren’t rooted in a desire to automate people out of jobs, they are rooted in market forces and bad leadership or management within organisations.
In fact, in the list of 95 companies I tracked from the article (some couldn’t be added due to a lack of concrete figures or reasons) only two mentioned Generative AI. One was pivoting towards it. Hiring more Gen AI-skilled individuals and letting go of other talents (A callback to the need to upskills). The other was Duolingo who cut 10% of their contractors in favour of using GPT models to carry out tasks.
As I say, this is admittedly an anecdotal sample and a snapshot in time. But it’s fair to say in 2024, two years after the popularisation of Gen AI, it’s not AI that has caused most redundancies but the common challenges that all businesses face. Cash flow and operations. If AI is going to impact the security of our role, we’re more likely to risk redundancy because it’s eating into the market share of the organisation we are working for than it is because it can do our jobs better than us. For example, the company Chegg had a 23% Reduction in workforce to return to growth. They work in the learning and education space which has been heavily impacted by the use of Gen AI.
I raise this because I believe that we need to be careful to not attribute blame in the wrong place. AI aren’t currently taking our jobs. Changing markets, trends and poor leadership are.
Conclusion
So quality engineering jobs aren’t going to disappear. But as stated at the start, they will change. That change will likely come more from the adoption of AI in the products we’re responsible for and the competitors that come onto the market that utilise them, rather than the tools that pose themselves as digital employees.
The real question we should be asking is how this change manifests. Will it be for the better or the worse? In Nicolas Carr’s The Glass Cage, he describes how roles are phased out rather than abruptly replaced in one fell swoop. Generative AI will offer the opportunity to automate parts of our roles and it will also open new opportunities and new skills to learn. It’s our job to ensure that we adapt in a way that means we continue to deliver value, responsibly, whilst learning to be effective in other areas of software development. Rather than let AI replace aspects of our work, we should strive to look for ways in which AI can augment our work.
This has already happened within other industries. In Rick Beato’s YouTube video How Auto-Tune DESTROYED Popular Music he shares how Auto-Tune as a tool that automated parts of the music production process paved the way for AI-generated music. He argues that we’re so used to listening to the smoothed-out pitches of vocals and instruments that have been treated by Auto-tune, we can no longer tell the difference between that and synthetically generated music. We face the same challenge as Quality engineers as well.
So the future of our roles is in our hands. Personally, if we want to continue to be successful quality engineers then I believe that the successful use of AI lies in a symbiosis of us and it. We want to be in a position in which we are looking at ways in which we can learn about and use AI to help enhance our abilities rather than make elements of our work redundant. It’s not an easy path to take. It takes time, effort and focus. But we each have a responsibility to continuously learn and grow in a way that supports this way of working.