The AI Area of Effect Model
The AI area of effect model describes the relationship between us and AI. Learn how it has recently been improved.
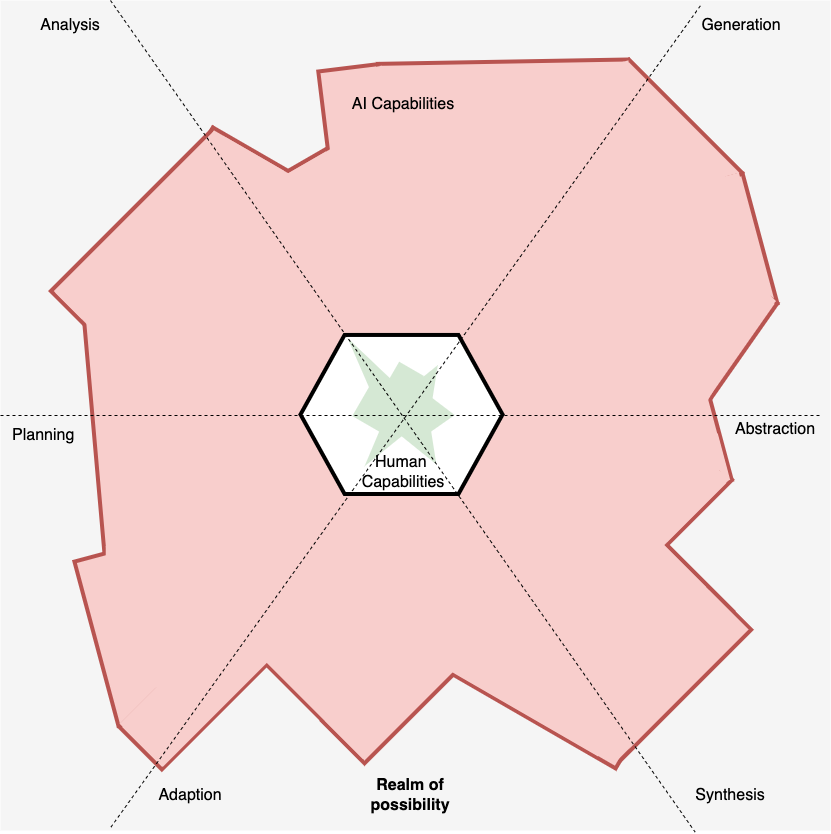
One of the goals of Software Testing with Generative AI was to advocate a mindset in which we use Generative AI to extend and enhance our abilities, rather than replace them. To do this I created what I call the area of effect model. This can be visualised as so:
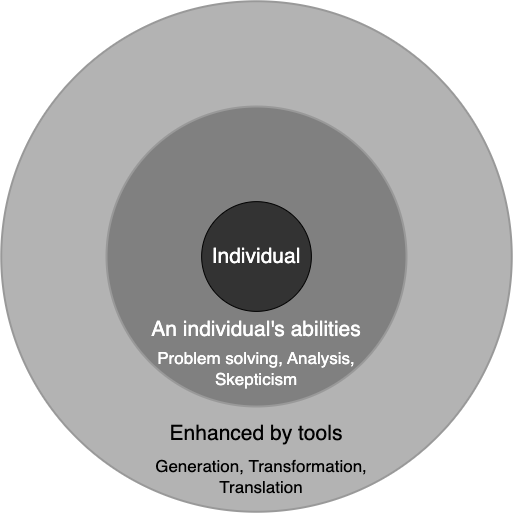
What the model communicates is that we as individuals are in the centre. Decisions and plans come from us because we are skilled in critical analysis, lateral thinking and planning. We then extend our abilities to carry out tasks by utilising what Generative AI is good at generation, transformation and translation of content. If we remove ourselves from the model, the tasks a Generative AI carries out become directionless. And if we were to remove the AI then our reach as engineers shrinks. A symbiosis of both is needed for success.
However, as is always the case, once a piece of work is completed you immediately start to see ways in which it can be improved upon which is exactly what happened after reading Co-Intelligence by Ethan Mollick over the holidays. So in this post, I’m going to explore how Mollick describes the capabilities of AI and how it inspired me to create version 2 of the area of effect model which is visualised as:
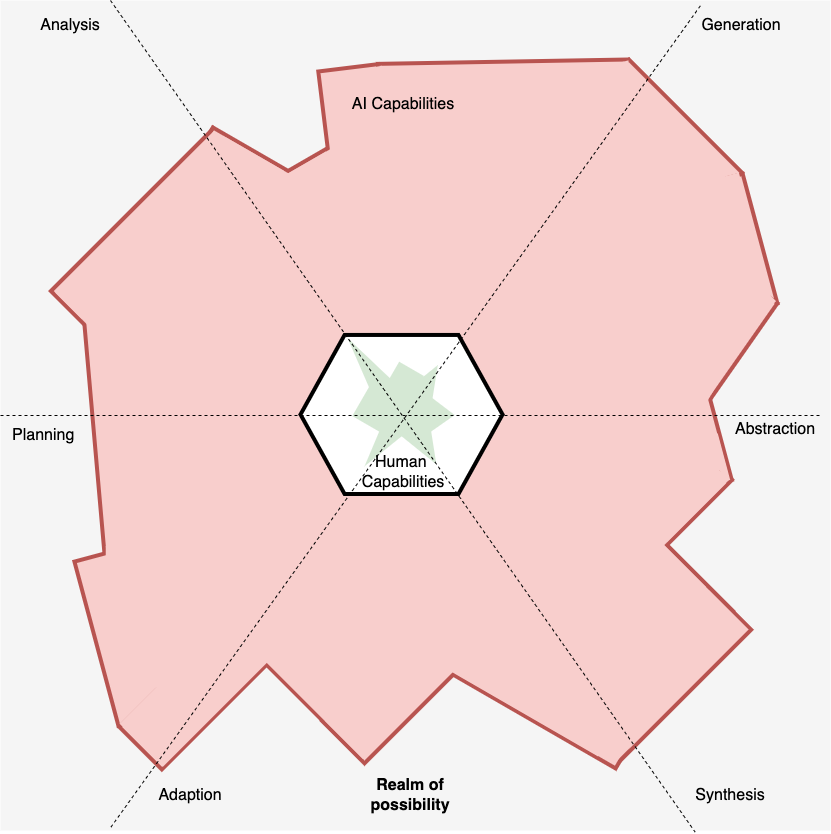
The Jagged frontier of AI
Co-Intelligence is an interesting book in which Mollick, a professor of management at Wharton, explores the impact of Generative AI on business and education. When reading literature on AI that has a positive slant on it I tend to lean into skepticism to balance out any potential hyperbole. But predictions aside, I found his models for explaining the power of Generative AI to be very informative and it helped me revise a model I use extensively in Software Testing with Generative AI, the area of effect model. So let’s explore a bit of Mollick’s work and how it can be used to improve our understanding of the relationship between Gen AI and ourselves.
In Co-Intelligence, Mollick discusses how Generative AI has the potential to help us with lots of different tasks but there is a limit or boundary to what it can do. The issue with this boundary is that it is not a clear, distinct one, but one that juts out or recedes in, depending on the task at hand:
Imagine a fortress wall, with some towers and battlements jutting out into the countryside, while others fold back towards the center of the castle. That wall is the capability of AI, and the further from the center, the harder the task. Everything inside the wall can be done by the AI, everything outside is hard for the AI to do.
To better understand this, Mollick created a visual representation in his blog post Centaurs and Cyborgs on the Jagged Frontier:
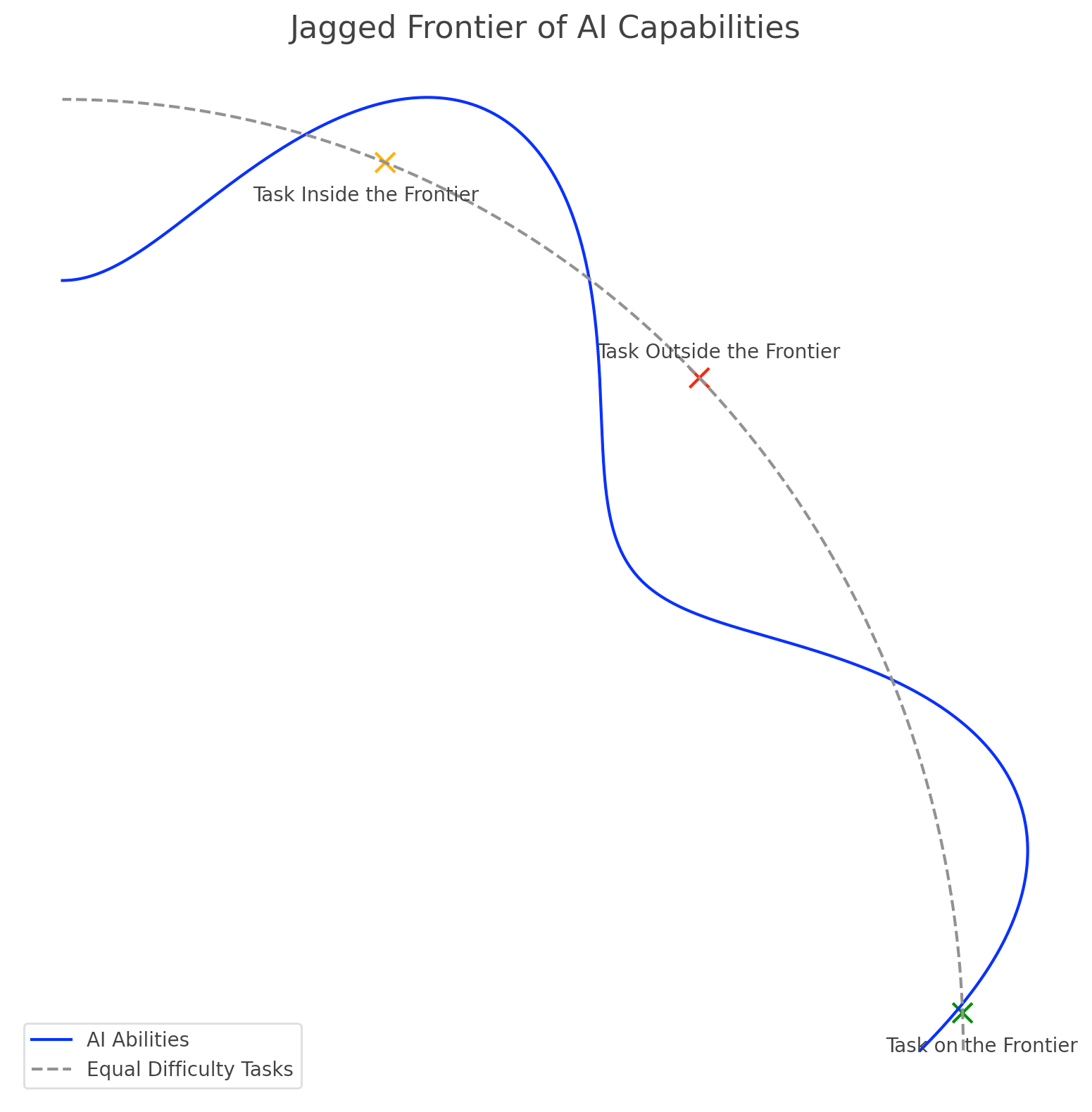
As we can see, the thick blue line represents potential tasks that Generative AI is capable of. Its waviness implies that it is better at some activities than others when compared to the dotted line of equally difficult tasks. So this model is useful because it helps us to appreciate that Gen AI has its uses in certain areas of our work, but not all.
Area of effect model version 2
So with the Jagged Frontier model in mind, I decided that the area of effect model could be improved on and arrived at version 2:
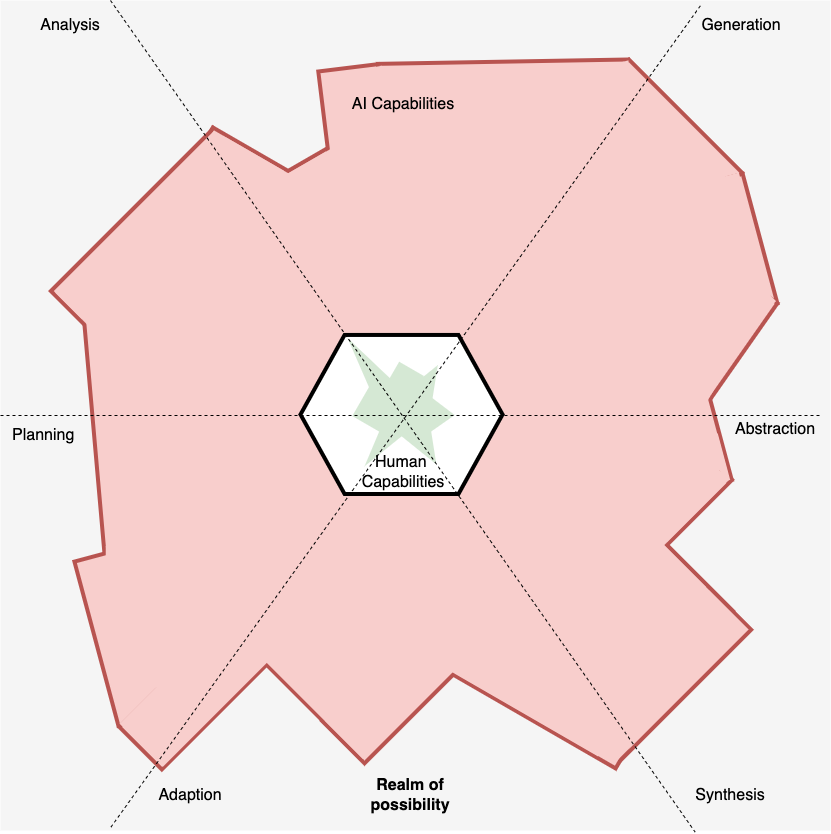
First, we start with the centre. When considering the Jagged Frontier it occurred to me that just as AI have strengths and weaknesses so do we as individuals. Therefore a spider diagram, a type of model that is used regularly to quantify an individual’s abilities, works well to highlight that we can benefit from using AI to bolster our weaker areas. The spider diagram concept is then extended in the form of dashed lines to demonstrate the different capabilities of AI. It’s worth mentioning that the proposed capabilities are by no means exhaustive and will likely need to be tweaked in a future iteration. But the idea is to use the lines to connect our abilities with AI capabilities. Finally, we have the red section that highlights the AI capabilities expanding our reach into the realm of possibility. Making it clear that not everything can or should be done with the use of AI.
I feel this new revised model combines the best of both the Jagged Frontier and the Area of effect. It brings to the fore both the relationship between us and AI but also shows that, as with all tools, there are limits to what we can and can’t do with them. Therefore, to get the most out of this model we must reflect on our strengths and weaknesses and determine where AI might help us, then experiment with these tools to better understand where they are useful and where they are not.
If you want to know more about the relationship between Software testing and AI, then please check out my new booking Software Testing with Generative AI.